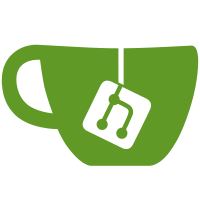
*实现agent ReAct与LLM chain自动切换逻辑✓ 聊天窗区分任务消息✓ 修复删除日程bug✓ 优化远程音频逻辑✓ 等待处理引入加载中效果✓ 优化prompt以解决日程任务递归调用问题✓ 修复一次性日程清除的bug✓
199 lines
7.5 KiB
Python
199 lines
7.5 KiB
Python
from langchain.embeddings.openai import OpenAIEmbeddings
|
||
from langchain.chat_models import ChatOpenAI
|
||
from langchain.memory import VectorStoreRetrieverMemory
|
||
import faiss
|
||
from langchain.docstore import InMemoryDocstore
|
||
from langchain.vectorstores import FAISS
|
||
from langchain.agents import AgentExecutor, Tool, ZeroShotAgent, initialize_agent, agent_types
|
||
from langchain.chains import LLMChain
|
||
from langchain.prompts import PromptTemplate
|
||
|
||
from agent.tools.MyTimer import MyTimer
|
||
from agent.tools.QueryTime import QueryTime
|
||
from agent.tools.Weather import Weather
|
||
from agent.tools.Calculator import Calculator
|
||
from agent.tools.CheckSensor import CheckSensor
|
||
from agent.tools.Switch import Switch
|
||
from agent.tools.Knowledge import Knowledge
|
||
from agent.tools.Say import Say
|
||
from agent.tools.QueryTimerDB import QueryTimerDB
|
||
from agent.tools.DeleteTimer import DeleteTimer
|
||
from agent.tools.GetSwitchLog import GetSwitchLog
|
||
from agent.tools.getOnRunLinkage import getOnRunLinkage
|
||
from agent.tools.SetChatStatus import SetChatStatus
|
||
|
||
|
||
import utils.config_util as utils
|
||
from core.content_db import Content_Db
|
||
from core import wsa_server
|
||
import os
|
||
|
||
|
||
|
||
class FayAgentCore():
|
||
def __init__(self):
|
||
|
||
utils.load_config()
|
||
os.environ['OPENAI_API_KEY'] = utils.key_gpt_api_key
|
||
#使用open ai embedding
|
||
embedding_size = 1536 # OpenAIEmbeddings 的维度
|
||
index = faiss.IndexFlatL2(embedding_size)
|
||
embedding_fn = OpenAIEmbeddings()
|
||
|
||
#创建llm
|
||
llm = ChatOpenAI(model="gpt-4-1106-preview", verbose=True)
|
||
|
||
#创建向量数据库
|
||
vectorstore = FAISS(embedding_fn, index, InMemoryDocstore({}), {})
|
||
|
||
# 创建记忆
|
||
retriever = vectorstore.as_retriever(search_kwargs=dict(k=2))
|
||
memory = VectorStoreRetrieverMemory(memory_key="chat_history", retriever=retriever)
|
||
|
||
# 保存基本信息到记忆
|
||
utils.load_config()
|
||
attr_info = ", ".join(f"{key}: {value}" for key, value in utils.config["attribute"].items())
|
||
memory.save_context({"input": "我的基本信息是?"}, {"output": attr_info})
|
||
|
||
#创建agent chain
|
||
my_timer = MyTimer()
|
||
query_time_tool = QueryTime()
|
||
weather_tool = Weather()
|
||
calculator_tool = Calculator()
|
||
check_sensor_tool = CheckSensor()
|
||
switch_tool = Switch()
|
||
knowledge_tool = Knowledge()
|
||
say_tool = Say()
|
||
query_timer_db_tool = QueryTimerDB()
|
||
delete_timer_tool = DeleteTimer()
|
||
get_switch_log = GetSwitchLog()
|
||
get_on_run_linkage = getOnRunLinkage()
|
||
set_chat_status_tool = SetChatStatus()
|
||
|
||
tools = [
|
||
Tool(
|
||
name=my_timer.name,
|
||
func=my_timer.run,
|
||
description=my_timer.description
|
||
),
|
||
Tool(
|
||
name=query_time_tool.name,
|
||
func=query_time_tool.run,
|
||
description=query_time_tool.description
|
||
),
|
||
Tool(
|
||
name=weather_tool.name,
|
||
func=weather_tool.run,
|
||
description=weather_tool.description
|
||
),
|
||
Tool(
|
||
name=calculator_tool.name,
|
||
func=calculator_tool.run,
|
||
description=calculator_tool.description
|
||
),
|
||
Tool(
|
||
name=check_sensor_tool.name,
|
||
func=check_sensor_tool.run,
|
||
description=check_sensor_tool.description
|
||
),
|
||
Tool(
|
||
name=switch_tool.name,
|
||
func=switch_tool.run,
|
||
description=switch_tool.description
|
||
),
|
||
Tool(
|
||
name=knowledge_tool.name,
|
||
func=knowledge_tool.run,
|
||
description=knowledge_tool.description
|
||
),
|
||
Tool(
|
||
name=say_tool.name,
|
||
func=say_tool.run,
|
||
description=say_tool.description
|
||
),
|
||
Tool(
|
||
name=query_timer_db_tool.name,
|
||
func=query_timer_db_tool.run,
|
||
description=query_timer_db_tool.description
|
||
),
|
||
Tool(
|
||
name=delete_timer_tool.name,
|
||
func=delete_timer_tool.run,
|
||
description=delete_timer_tool.description
|
||
),
|
||
Tool(
|
||
name=get_switch_log.name,
|
||
func=get_switch_log.run,
|
||
description=get_switch_log.description
|
||
),
|
||
Tool(
|
||
name=get_on_run_linkage.name,
|
||
func=get_on_run_linkage.run,
|
||
description=get_on_run_linkage.description
|
||
),
|
||
Tool(
|
||
name=set_chat_status_tool.name,
|
||
func=set_chat_status_tool.run,
|
||
description=set_chat_status_tool.description
|
||
),
|
||
]
|
||
|
||
#agent用于执行任务
|
||
self.agent = initialize_agent(agent_types=agent_types.AgentType.CHAT_CONVERSATIONAL_REACT_DESCRIPTION,
|
||
tools=tools, llm=llm, verbose=True,
|
||
max_history=5,
|
||
memory=memory, handle_parsing_errors=True)
|
||
|
||
#创建llm chain用于聊天
|
||
tools_prompt = "["
|
||
tool_names = [tool.name for tool in tools if tool.name != set_chat_status_tool.name and tool.name != say_tool.name]
|
||
tools_prompt += "、".join(tool_names) + "]"
|
||
template = """你是一个智慧农业实验箱里的ai,你的责任是陪伴主人生活、工作,以及协助主人打理好农业种植箱里的农作物.现在主人正在和你聊天,若你在聊天过程中感觉到主人想使用以下工具,请按“agent:'主人刚刚的说话'”这样的格式回复,否则请直接回复我文字内容。工具如下:
|
||
""" + tools_prompt +"""
|
||
{chat_history}
|
||
Human: {human_input}
|
||
AI:"""
|
||
prompt = PromptTemplate(
|
||
input_variables=["chat_history", "human_input"], template=template
|
||
)
|
||
self.llm_chain = LLMChain(
|
||
llm=llm,
|
||
prompt=prompt,
|
||
verbose=True,
|
||
memory=memory
|
||
)
|
||
|
||
self.is_chat = False#聊天状态
|
||
|
||
|
||
def run(self, input_text):
|
||
#消息保存
|
||
contentdb = Content_Db()
|
||
contentdb.add_content('member', 'agent', input_text.replace('主人语音说了:', '').replace('主人文字说了:', ''))
|
||
wsa_server.get_web_instance().add_cmd({"panelReply": {"type":"member","content":input_text.replace('主人语音说了:', '').replace('主人文字说了:', '')}})
|
||
result = ""
|
||
try:
|
||
#判断执行聊天模式还是agent模式,双模式在运行过程中会主动切换
|
||
if self.is_chat:
|
||
result = self.llm_chain.predict(human_input=input_text.replace('主人语音说了:', '').replace('主人文字说了:', ''))
|
||
if "agent:" in result.lower() or not self.is_chat:
|
||
print(result)
|
||
print(self.is_chat)
|
||
self.is_chat = False
|
||
input_text = result if result.lower().replace("agent:", "") else input_text
|
||
result = self.agent.run(input_text)
|
||
except Exception as e:
|
||
print(e)
|
||
result = "执行完毕" if result is None or result == "N/A" else result
|
||
|
||
|
||
#消息保存
|
||
contentdb.add_content('fay','agent', result)
|
||
wsa_server.get_web_instance().add_cmd({"panelReply": {"type":"fay","content":result}})
|
||
|
||
return result
|
||
|
||
if __name__ == "__main__":
|
||
agent = FayAgentCore()
|
||
print(agent.run("你好"))
|