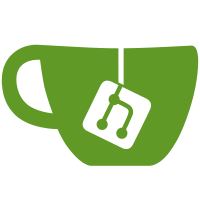
- 全新ui - 全面优化websocket逻辑,提高数字人和ui连接的稳定性及资源开销 - 全面优化唤醒逻辑,提供稳定的普通唤醒模式和前置词唤醒模式 - 优化拾音质量,支持多声道麦克风拾音 - 优化自动播放服务器的对接机制,提供稳定和兼容旧版ue工程的对接模式 - 数字人接口输出机器人表情,以适应新fay ui及单片机的数字人表情输出 - 使用更高级的音频时长计算方式,可以更精准控制音频播放完成后的逻辑 - 修复点击关闭按钮会导致程序退出的bug - 修复没有麦克风的设备开启麦克风会出错的问题 - 为服务器主机地址提供配置项,以方便服务器部署
651 lines
22 KiB
Python
651 lines
22 KiB
Python
"""
|
||
This script implements an API for the ChatGLM3-6B model,
|
||
formatted similarly to OpenAI's API (https://platform.openai.com/docs/api-reference/chat).
|
||
It's designed to be run as a web server using FastAPI and uvicorn,
|
||
making the ChatGLM3-6B model accessible through OpenAI Client.
|
||
|
||
Key Components and Features:
|
||
- Model and Tokenizer Setup: Configures the model and tokenizer paths and loads them.
|
||
- FastAPI Configuration: Sets up a FastAPI application with CORS middleware for handling cross-origin requests.
|
||
- API Endpoints:
|
||
- "/v1/models": Lists the available models, specifically ChatGLM3-6B.
|
||
- "/v1/chat/completions": Processes chat completion requests with options for streaming and regular responses.
|
||
- "/v1/embeddings": Processes Embedding request of a list of text inputs.
|
||
- Token Limit Caution: In the OpenAI API, 'max_tokens' is equivalent to HuggingFace's 'max_new_tokens', not 'max_length'.
|
||
For instance, setting 'max_tokens' to 8192 for a 6b model would result in an error due to the model's inability to output
|
||
that many tokens after accounting for the history and prompt tokens.
|
||
- Stream Handling and Custom Functions: Manages streaming responses and custom function calls within chat responses.
|
||
- Pydantic Models: Defines structured models for requests and responses, enhancing API documentation and type safety.
|
||
- Main Execution: Initializes the model and tokenizer, and starts the FastAPI app on the designated host and port.
|
||
|
||
Note:
|
||
This script doesn't include the setup for special tokens or multi-GPU support by default.
|
||
Users need to configure their special tokens and can enable multi-GPU support as per the provided instructions.
|
||
Embedding Models only support in One GPU.
|
||
|
||
"""
|
||
|
||
import os
|
||
import time
|
||
import tiktoken
|
||
import torch
|
||
import uvicorn
|
||
import json, datetime
|
||
|
||
from fastapi import FastAPI, HTTPException, Request, Response
|
||
from fastapi.middleware.cors import CORSMiddleware
|
||
|
||
from contextlib import asynccontextmanager
|
||
from typing import List, Literal, Optional, Union
|
||
|
||
from loguru import logger
|
||
from pydantic import BaseModel, Field
|
||
from transformers import AutoTokenizer, AutoModel
|
||
from utils import process_response, generate_chatglm3, generate_stream_chatglm3
|
||
from sentence_transformers import SentenceTransformer
|
||
|
||
from sse_starlette.sse import EventSourceResponse
|
||
|
||
|
||
# Set up limit request time
|
||
EventSourceResponse.DEFAULT_PING_INTERVAL = 1000
|
||
|
||
# set LLM path
|
||
MODEL_PATH = os.environ.get('MODEL_PATH', 'THUDM/chatglm3-6b')
|
||
TOKENIZER_PATH = os.environ.get("TOKENIZER_PATH", MODEL_PATH)
|
||
|
||
# set Embedding Model path
|
||
EMBEDDING_PATH = os.environ.get('EMBEDDING_PATH', 'BAAI/bge-large-zh-v1.5')
|
||
|
||
|
||
@asynccontextmanager
|
||
async def lifespan(app: FastAPI):
|
||
yield
|
||
if torch.cuda.is_available():
|
||
torch.cuda.empty_cache()
|
||
torch.cuda.ipc_collect()
|
||
|
||
|
||
app = FastAPI(lifespan=lifespan)
|
||
|
||
app.add_middleware(
|
||
CORSMiddleware,
|
||
allow_origins=["*"],
|
||
allow_credentials=True,
|
||
allow_methods=["*"],
|
||
allow_headers=["*"],
|
||
)
|
||
|
||
|
||
class ModelCard(BaseModel):
|
||
id: str
|
||
object: str = "model"
|
||
created: int = Field(default_factory=lambda: int(time.time()))
|
||
owned_by: str = "owner"
|
||
root: Optional[str] = None
|
||
parent: Optional[str] = None
|
||
permission: Optional[list] = None
|
||
|
||
|
||
class ModelList(BaseModel):
|
||
object: str = "list"
|
||
data: List[ModelCard] = []
|
||
|
||
|
||
class FunctionCallResponse(BaseModel):
|
||
name: Optional[str] = None
|
||
arguments: Optional[str] = None
|
||
|
||
|
||
class ChatMessage(BaseModel):
|
||
role: Literal["user", "assistant", "system", "function"]
|
||
content: str = None
|
||
name: Optional[str] = None
|
||
function_call: Optional[FunctionCallResponse] = None
|
||
|
||
|
||
class DeltaMessage(BaseModel):
|
||
role: Optional[Literal["user", "assistant", "system"]] = None
|
||
content: Optional[str] = None
|
||
function_call: Optional[FunctionCallResponse] = None
|
||
|
||
|
||
## for Embedding
|
||
class EmbeddingRequest(BaseModel):
|
||
input: List[str]
|
||
model: str
|
||
|
||
|
||
class CompletionUsage(BaseModel):
|
||
prompt_tokens: int
|
||
completion_tokens: int
|
||
total_tokens: int
|
||
|
||
|
||
class EmbeddingResponse(BaseModel):
|
||
data: list
|
||
model: str
|
||
object: str
|
||
usage: CompletionUsage
|
||
|
||
|
||
# for ChatCompletionRequest
|
||
|
||
class UsageInfo(BaseModel):
|
||
prompt_tokens: int = 0
|
||
total_tokens: int = 0
|
||
completion_tokens: Optional[int] = 0
|
||
|
||
|
||
class ChatCompletionRequest(BaseModel):
|
||
model: str
|
||
messages: List[ChatMessage]
|
||
temperature: Optional[float] = 0.8
|
||
top_p: Optional[float] = 0.8
|
||
max_tokens: Optional[int] = None
|
||
stream: Optional[bool] = False
|
||
tools: Optional[Union[dict, List[dict]]] = None
|
||
repetition_penalty: Optional[float] = 1.1
|
||
|
||
|
||
class ChatCompletionResponseChoice(BaseModel):
|
||
index: int
|
||
message: ChatMessage
|
||
finish_reason: Literal["stop", "length", "function_call"]
|
||
|
||
|
||
class ChatCompletionResponseStreamChoice(BaseModel):
|
||
delta: DeltaMessage
|
||
finish_reason: Optional[Literal["stop", "length", "function_call"]]
|
||
index: int
|
||
|
||
|
||
class ChatCompletionResponse(BaseModel):
|
||
model: str
|
||
id: str
|
||
object: Literal["chat.completion", "chat.completion.chunk"]
|
||
choices: List[Union[ChatCompletionResponseChoice, ChatCompletionResponseStreamChoice]]
|
||
created: Optional[int] = Field(default_factory=lambda: int(time.time()))
|
||
usage: Optional[UsageInfo] = None
|
||
|
||
|
||
@app.get("/health")
|
||
async def health() -> Response:
|
||
"""Health check."""
|
||
return Response(status_code=200)
|
||
|
||
@app.post("/v1/completions")
|
||
async def create_item1(request: Request):
|
||
global model, tokenizer
|
||
|
||
start_time = datetime.datetime.now()
|
||
json_post_raw = await request.json()
|
||
json_post = json.dumps(json_post_raw)
|
||
json_post_list = json.loads(json_post)
|
||
prompt = json_post_list.get('prompt')
|
||
history = json_post_list.get('history')
|
||
print("===============history=========================")
|
||
print(history)
|
||
print("===============history=========================")
|
||
max_length = json_post_list.get('max_length')
|
||
top_p = json_post_list.get('top_p')
|
||
temperature = json_post_list.get('temperature')
|
||
response, history = model.chat(tokenizer,
|
||
prompt,
|
||
history=history,
|
||
max_length=max_length if max_length else 2048,
|
||
top_p=top_p if top_p else 0.7,
|
||
temperature=temperature if temperature else 0.95)
|
||
now = datetime.datetime.now()
|
||
time = now.strftime("%Y-%m-%d %H:%M:%S")
|
||
answer = {
|
||
"response": response,
|
||
"history": history,
|
||
"status": 200,
|
||
"time": time
|
||
}
|
||
|
||
time_difference = now-start_time
|
||
print("消耗时间差为:", time_difference)
|
||
log = "[" + time + "] " + '", prompt:"' + prompt + '", response:"' + repr(response) + '"'
|
||
print(log)
|
||
return answer
|
||
|
||
@app.post("/v1/chat")
|
||
async def create_item3(request: Request):
|
||
global model, tokenizer
|
||
|
||
start_time = datetime.datetime.now()
|
||
json_post_raw = await request.json()
|
||
json_post = json.dumps(json_post_raw)
|
||
json_post_list = json.loads(json_post)
|
||
prompt = json_post_list.get('prompt')
|
||
historys = json_post_list.get('history')
|
||
print("===============historys=========================")
|
||
print(historys)
|
||
print("===============historys=========================")
|
||
history = []
|
||
# if historys is not None:
|
||
# for string in historys:
|
||
# # 打印字符串
|
||
# print(string)
|
||
# # for his in string:
|
||
# # print(his)
|
||
# for his in string:
|
||
# dialogue = []
|
||
# dialogue.append('user',his)
|
||
# history.append(dialogue)
|
||
if historys is not None:
|
||
for string in historys:
|
||
# 打印字符串
|
||
print(string)
|
||
# for his in string:
|
||
# print(his)
|
||
i = 0
|
||
for his in string:
|
||
print(his)
|
||
if i==0:
|
||
dialogue={
|
||
"role": "user",
|
||
"content": his
|
||
}
|
||
elif i==1:
|
||
dialogue={
|
||
"role": "assistant",
|
||
"content": his
|
||
}
|
||
history.append(dialogue)
|
||
i = 1
|
||
current = {
|
||
"role": "user",
|
||
"content": prompt
|
||
}
|
||
history.append(current)
|
||
|
||
|
||
# log22 = "[" + history + "] "
|
||
print("===============history=========================")
|
||
print(history)
|
||
print("===============history=========================")
|
||
|
||
max_length = json_post_list.get('max_length')
|
||
top_p = json_post_list.get('top_p')
|
||
temperature = json_post_list.get('temperature')
|
||
response, history = model.chat(tokenizer,
|
||
prompt,
|
||
history=history,
|
||
max_length=max_length if max_length else 2048,
|
||
top_p=top_p if top_p else 0.7,
|
||
temperature=temperature if temperature else 0.95)
|
||
now = datetime.datetime.now()
|
||
time = now.strftime("%Y-%m-%d %H:%M:%S")
|
||
answer = {
|
||
"response": response,
|
||
"history": history,
|
||
"status": 200,
|
||
"time": time
|
||
}
|
||
|
||
time_difference = now-start_time
|
||
print("消耗时间差为:", time_difference)
|
||
log = "[" + time + "] " + '", prompt:"' + prompt + '", response:"' + repr(response) + '"'
|
||
print(log)
|
||
return answer
|
||
|
||
@app.post("/v1/embeddings", response_model=EmbeddingResponse)
|
||
async def get_embeddings(request: EmbeddingRequest):
|
||
embeddings = [embedding_model.encode(text) for text in request.input]
|
||
embeddings = [embedding.tolist() for embedding in embeddings]
|
||
|
||
def num_tokens_from_string(string: str) -> int:
|
||
"""
|
||
Returns the number of tokens in a text string.
|
||
use cl100k_base tokenizer
|
||
"""
|
||
encoding = tiktoken.get_encoding('cl100k_base')
|
||
num_tokens = len(encoding.encode(string))
|
||
return num_tokens
|
||
|
||
response = {
|
||
"data": [
|
||
{
|
||
"object": "embedding",
|
||
"embedding": embedding,
|
||
"index": index
|
||
}
|
||
for index, embedding in enumerate(embeddings)
|
||
],
|
||
"model": request.model,
|
||
"object": "list",
|
||
"usage": CompletionUsage(
|
||
prompt_tokens=sum(len(text.split()) for text in request.input),
|
||
completion_tokens=0,
|
||
total_tokens=sum(num_tokens_from_string(text) for text in request.input),
|
||
)
|
||
}
|
||
return response
|
||
|
||
|
||
@app.get("/v1/models", response_model=ModelList)
|
||
async def list_models():
|
||
model_card = ModelCard(
|
||
id="chatglm3-6b"
|
||
)
|
||
return ModelList(
|
||
data=[model_card]
|
||
)
|
||
|
||
|
||
@app.post("/v1/chat/completions", response_model=ChatCompletionResponse)
|
||
async def create_chat_completion(request: ChatCompletionRequest):
|
||
global model, tokenizer
|
||
|
||
if len(request.messages) < 1 or request.messages[-1].role == "assistant":
|
||
raise HTTPException(status_code=400, detail="Invalid request")
|
||
|
||
gen_params = dict(
|
||
messages=request.messages,
|
||
temperature=request.temperature,
|
||
top_p=request.top_p,
|
||
max_tokens=request.max_tokens or 1024,
|
||
echo=False,
|
||
stream=request.stream,
|
||
repetition_penalty=request.repetition_penalty,
|
||
tools=request.tools,
|
||
)
|
||
logger.debug(f"==== request ====\n{gen_params}")
|
||
|
||
if request.stream:
|
||
|
||
# Use the stream mode to read the first few characters, if it is not a function call, direct stram output
|
||
predict_stream_generator = predict_stream(request.model, gen_params)
|
||
output = next(predict_stream_generator)
|
||
if not contains_custom_function(output):
|
||
return EventSourceResponse(predict_stream_generator, media_type="text/event-stream")
|
||
|
||
# Obtain the result directly at one time and determine whether tools needs to be called.
|
||
logger.debug(f"First result output:\n{output}")
|
||
|
||
function_call = None
|
||
if output and request.tools:
|
||
try:
|
||
function_call = process_response(output, use_tool=True)
|
||
except:
|
||
logger.warning("Failed to parse tool call")
|
||
|
||
# CallFunction
|
||
if isinstance(function_call, dict):
|
||
function_call = FunctionCallResponse(**function_call)
|
||
|
||
"""
|
||
In this demo, we did not register any tools.
|
||
You can use the tools that have been implemented in our `tools_using_demo` and implement your own streaming tool implementation here.
|
||
Similar to the following method:
|
||
function_args = json.loads(function_call.arguments)
|
||
tool_response = dispatch_tool(tool_name: str, tool_params: dict)
|
||
"""
|
||
tool_response = ""
|
||
|
||
if not gen_params.get("messages"):
|
||
gen_params["messages"] = []
|
||
|
||
gen_params["messages"].append(ChatMessage(
|
||
role="assistant",
|
||
content=output,
|
||
))
|
||
gen_params["messages"].append(ChatMessage(
|
||
role="function",
|
||
name=function_call.name,
|
||
content=tool_response,
|
||
))
|
||
|
||
# Streaming output of results after function calls
|
||
generate = predict(request.model, gen_params)
|
||
return EventSourceResponse(generate, media_type="text/event-stream")
|
||
|
||
else:
|
||
# Handled to avoid exceptions in the above parsing function process.
|
||
generate = parse_output_text(request.model, output)
|
||
return EventSourceResponse(generate, media_type="text/event-stream")
|
||
|
||
# Here is the handling of stream = False
|
||
response = generate_chatglm3(model, tokenizer, gen_params)
|
||
|
||
# Remove the first newline character
|
||
if response["text"].startswith("\n"):
|
||
response["text"] = response["text"][1:]
|
||
response["text"] = response["text"].strip()
|
||
|
||
usage = UsageInfo()
|
||
function_call, finish_reason = None, "stop"
|
||
if request.tools:
|
||
try:
|
||
function_call = process_response(response["text"], use_tool=True)
|
||
except:
|
||
logger.warning("Failed to parse tool call, maybe the response is not a tool call or have been answered.")
|
||
|
||
if isinstance(function_call, dict):
|
||
finish_reason = "function_call"
|
||
function_call = FunctionCallResponse(**function_call)
|
||
|
||
message = ChatMessage(
|
||
role="assistant",
|
||
content=response["text"],
|
||
function_call=function_call if isinstance(function_call, FunctionCallResponse) else None,
|
||
)
|
||
|
||
logger.debug(f"==== message ====\n{message}")
|
||
|
||
choice_data = ChatCompletionResponseChoice(
|
||
index=0,
|
||
message=message,
|
||
finish_reason=finish_reason,
|
||
)
|
||
task_usage = UsageInfo.model_validate(response["usage"])
|
||
for usage_key, usage_value in task_usage.model_dump().items():
|
||
setattr(usage, usage_key, getattr(usage, usage_key) + usage_value)
|
||
|
||
return ChatCompletionResponse(
|
||
model=request.model,
|
||
id="", # for open_source model, id is empty
|
||
choices=[choice_data],
|
||
object="chat.completion",
|
||
usage=usage
|
||
)
|
||
|
||
|
||
async def predict(model_id: str, params: dict):
|
||
global model, tokenizer
|
||
|
||
choice_data = ChatCompletionResponseStreamChoice(
|
||
index=0,
|
||
delta=DeltaMessage(role="assistant"),
|
||
finish_reason=None
|
||
)
|
||
chunk = ChatCompletionResponse(model=model_id, id="", choices=[choice_data], object="chat.completion.chunk")
|
||
yield "{}".format(chunk.model_dump_json(exclude_unset=True))
|
||
|
||
previous_text = ""
|
||
for new_response in generate_stream_chatglm3(model, tokenizer, params):
|
||
decoded_unicode = new_response["text"]
|
||
delta_text = decoded_unicode[len(previous_text):]
|
||
previous_text = decoded_unicode
|
||
|
||
finish_reason = new_response["finish_reason"]
|
||
if len(delta_text) == 0 and finish_reason != "function_call":
|
||
continue
|
||
|
||
function_call = None
|
||
if finish_reason == "function_call":
|
||
try:
|
||
function_call = process_response(decoded_unicode, use_tool=True)
|
||
except:
|
||
logger.warning(
|
||
"Failed to parse tool call, maybe the response is not a tool call or have been answered.")
|
||
|
||
if isinstance(function_call, dict):
|
||
function_call = FunctionCallResponse(**function_call)
|
||
|
||
delta = DeltaMessage(
|
||
content=delta_text,
|
||
role="assistant",
|
||
function_call=function_call if isinstance(function_call, FunctionCallResponse) else None,
|
||
)
|
||
|
||
choice_data = ChatCompletionResponseStreamChoice(
|
||
index=0,
|
||
delta=delta,
|
||
finish_reason=finish_reason
|
||
)
|
||
chunk = ChatCompletionResponse(
|
||
model=model_id,
|
||
id="",
|
||
choices=[choice_data],
|
||
object="chat.completion.chunk"
|
||
)
|
||
yield "{}".format(chunk.model_dump_json(exclude_unset=True))
|
||
|
||
choice_data = ChatCompletionResponseStreamChoice(
|
||
index=0,
|
||
delta=DeltaMessage(),
|
||
finish_reason="stop"
|
||
)
|
||
chunk = ChatCompletionResponse(
|
||
model=model_id,
|
||
id="",
|
||
choices=[choice_data],
|
||
object="chat.completion.chunk"
|
||
)
|
||
yield "{}".format(chunk.model_dump_json(exclude_unset=True))
|
||
yield '[DONE]'
|
||
|
||
|
||
def predict_stream(model_id, gen_params):
|
||
"""
|
||
The function call is compatible with stream mode output.
|
||
|
||
The first seven characters are determined.
|
||
If not a function call, the stream output is directly generated.
|
||
Otherwise, the complete character content of the function call is returned.
|
||
|
||
:param model_id:
|
||
:param gen_params:
|
||
:return:
|
||
"""
|
||
output = ""
|
||
is_function_call = False
|
||
has_send_first_chunk = False
|
||
for new_response in generate_stream_chatglm3(model, tokenizer, gen_params):
|
||
decoded_unicode = new_response["text"]
|
||
delta_text = decoded_unicode[len(output):]
|
||
output = decoded_unicode
|
||
|
||
# When it is not a function call and the character length is> 7,
|
||
# try to judge whether it is a function call according to the special function prefix
|
||
if not is_function_call and len(output) > 7:
|
||
|
||
# Determine whether a function is called
|
||
is_function_call = contains_custom_function(output)
|
||
if is_function_call:
|
||
continue
|
||
|
||
# Non-function call, direct stream output
|
||
finish_reason = new_response["finish_reason"]
|
||
|
||
# Send an empty string first to avoid truncation by subsequent next() operations.
|
||
if not has_send_first_chunk:
|
||
message = DeltaMessage(
|
||
content="",
|
||
role="assistant",
|
||
function_call=None,
|
||
)
|
||
choice_data = ChatCompletionResponseStreamChoice(
|
||
index=0,
|
||
delta=message,
|
||
finish_reason=finish_reason
|
||
)
|
||
chunk = ChatCompletionResponse(
|
||
model=model_id,
|
||
id="",
|
||
choices=[choice_data],
|
||
created=int(time.time()),
|
||
object="chat.completion.chunk"
|
||
)
|
||
yield "{}".format(chunk.model_dump_json(exclude_unset=True))
|
||
|
||
send_msg = delta_text if has_send_first_chunk else output
|
||
has_send_first_chunk = True
|
||
message = DeltaMessage(
|
||
content=send_msg,
|
||
role="assistant",
|
||
function_call=None,
|
||
)
|
||
choice_data = ChatCompletionResponseStreamChoice(
|
||
index=0,
|
||
delta=message,
|
||
finish_reason=finish_reason
|
||
)
|
||
chunk = ChatCompletionResponse(
|
||
model=model_id,
|
||
id="",
|
||
choices=[choice_data],
|
||
created=int(time.time()),
|
||
object="chat.completion.chunk"
|
||
)
|
||
yield "{}".format(chunk.model_dump_json(exclude_unset=True))
|
||
|
||
if is_function_call:
|
||
yield output
|
||
else:
|
||
yield '[DONE]'
|
||
|
||
|
||
async def parse_output_text(model_id: str, value: str):
|
||
"""
|
||
Directly output the text content of value
|
||
|
||
:param model_id:
|
||
:param value:
|
||
:return:
|
||
"""
|
||
choice_data = ChatCompletionResponseStreamChoice(
|
||
index=0,
|
||
delta=DeltaMessage(role="assistant", content=value),
|
||
finish_reason=None
|
||
)
|
||
chunk = ChatCompletionResponse(model=model_id, id="", choices=[choice_data], object="chat.completion.chunk")
|
||
yield "{}".format(chunk.model_dump_json(exclude_unset=True))
|
||
|
||
choice_data = ChatCompletionResponseStreamChoice(
|
||
index=0,
|
||
delta=DeltaMessage(),
|
||
finish_reason="stop"
|
||
)
|
||
chunk = ChatCompletionResponse(model=model_id, id="", choices=[choice_data], object="chat.completion.chunk")
|
||
yield "{}".format(chunk.model_dump_json(exclude_unset=True))
|
||
yield '[DONE]'
|
||
|
||
|
||
def contains_custom_function(value: str) -> bool:
|
||
"""
|
||
Determine whether 'function_call' according to a special function prefix.
|
||
|
||
For example, the functions defined in "tools_using_demo/tool_register.py" are all "get_xxx" and start with "get_"
|
||
|
||
[Note] This is not a rigorous judgment method, only for reference.
|
||
|
||
:param value:
|
||
:return:
|
||
"""
|
||
return value and 'get_' in value
|
||
|
||
|
||
if __name__ == "__main__":
|
||
# Load LLM
|
||
tokenizer = AutoTokenizer.from_pretrained(TOKENIZER_PATH, trust_remote_code=True)
|
||
model = AutoModel.from_pretrained(MODEL_PATH, trust_remote_code=True, device_map="auto").eval()
|
||
|
||
# load Embedding
|
||
embedding_model = SentenceTransformer(EMBEDDING_PATH, device="cuda")
|
||
uvicorn.run(app, host='0.0.0.0', port=8000, workers=1)
|