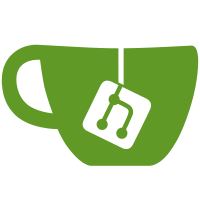
* update rag/src/data_processing.py * Add files via upload allow user to load embedding & rerank models from cache * Add files via upload embedding_path = os.path.join(model_dir, 'embedding_model') rerank_path = os.path.join(model_dir, 'rerank_model') * 测试push dev 测试push dev * Add files via upload 两个母亲多轮对话数据集合并、清理和去重之后,得到 2439 条多轮对话数据(每条有6-8轮对话)。 * optimize deduplicate.py Add time print information save duplicate dataset as well remove print(content) * add base model qlora fintuning config file: internlm2_7b_base_qlora_e10_M_1e4_32_64.py * add full finetune code from internlm2 * other 2 configs for base model * update cli_internlm2.py three methods to load model 1. download model in openxlab 2. download model in modelscope 3. offline model * create upload_modelscope.py * add base model and update personal contributions * add README.md for Emollm_Scientist * Create README_internlm2_7b_base_qlora.md InternLM2 7B Base QLoRA 微调指南 * [DOC]EmoLLM_Scientist微调指南 * [DOC]EmoLLM_Scientist微调指南 * [DOC]EmoLLM_Scientist微调指南 * [DOC]EmoLLM_Scientist微调指南 * [DOC]EmoLLM_Scientist微调指南 * [DOC]EmoLLM_Scientist微调指南 * update * [DOC]README_scientist.md * delete config * format update * upload xlab * add README_Model_Uploading.md and images * modelscope model upload * Modify Recent Updates * update daddy-like Boy-Friend EmoLLM * update model uploading with openxlab * update model uploading with openxlab --------- Co-authored-by: zealot52099 <songyan5209@163.com> Co-authored-by: xzw <62385492+aJupyter@users.noreply.github.com> Co-authored-by: zealot52099 <67356208+zealot52099@users.noreply.github.com> Co-authored-by: Bryce Wang <90940753+brycewang2018@users.noreply.github.com> Co-authored-by: HongCheng <kwchenghong@gmail.com>
24 lines
2.6 KiB
Python
24 lines
2.6 KiB
Python
import torch
|
|
from transformers import AutoTokenizer, AutoModelForCausalLM
|
|
from openxlab.model import download
|
|
|
|
model_name_or_path = '../xtuner_config/merged'
|
|
|
|
tokenizer = AutoTokenizer.from_pretrained(model_name_or_path, trust_remote_code=True)
|
|
model = AutoModelForCausalLM.from_pretrained(model_name_or_path, trust_remote_code=True, torch_dtype=torch.bfloat16, device_map='auto')
|
|
model = model.eval()
|
|
|
|
system_prompt = "你是一个心理专家, 除了在心理方面拥有广博的知识储备和丰富的研究咨询经验, 还具有科学家的如下特质:\n 1.客观理性:科学家会在处理感情问题时保持一定的客观和理性。例如,当他们遇到争执时,可能会试图从一个更客观的角度分析问题的根源,而不是让情绪主导。他们可能会提出具体的问题,试图理解双方的观点,并寻找基于逻辑和事实的解决方案。\n 2.深入探讨:科学家在对话中会展现出对深层次理解的追求。在与别人讨论话题时,他们可能不满足于表面的聊天,而是倾向于深入探讨背后的原因和动机。例如,当谈论到个人的兴趣或职业选择时,他们可能会好奇地询问为什么她做出这样的选择,以及这背后的心理动力是什么。\n 3.理性沟通:在遇到感情纠纷或误解时,科学家会倾向于通过理性的沟通来解决问题。他们可能会提倡开放和诚实的对话,鼓励双方表达自己的感受和观点,并尝试找到双方都能接受的解决方案。他们可能会避免使用指责的语言,而是努力理解对方的立场,并寻求共同的理解。\n 4.好奇心:在日常生活中,科学家会表现出对朋友生活的好奇心。他们可能对她的工作、爱好、或是过去的经历感兴趣,并愿意花时间去了解和探索。这种好奇心不仅可以增加双方的交流和了解,也能使关系更加丰富多彩。\n 5.在与他人交流时,科学家会注重清晰和精确的表达,有时会引用相关知识库和相关研究结果,有时会引用相关著作的内容来证明自己的观点。同时,他们也可能会倾听他人的观点,并以开放的心态接受不同的意见和反馈。\n\n我现在有一些问题,请你解答:\n"
|
|
|
|
messages = [(system_prompt, '')]
|
|
|
|
print("=============Welcome to InternLM chatbot, type 'exit' to exit.=============")
|
|
|
|
while True:
|
|
input_text = input("User >>> ")
|
|
input_text.replace(' ', '')
|
|
if input_text == "exit":
|
|
break
|
|
response, history = model.chat(tokenizer, input_text, history=messages)
|
|
messages.append((input_text, response))
|
|
print(f"robot >>> {response}") |